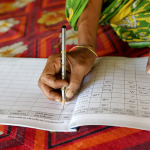
By Shaida Badiee and Claire Melamed
If there was a competition for the most repeated and least reliable statistic in the development business, then the oft-repeated ‘fact’ that 70% of the world’s poor are women might just win it. It might be true, it might not. But, shockingly and surprisingly given how often it’s used, there’s not really any way of knowing if it’s true.
The ubiquity and uncertainty of the 70% figure tells us two things about the data revolution. Firstly, that people want good data on gender – the over use of this dubious statistic indicates a demand out there that needs to be filled. Secondly, that at the moment we don’t have it – in many countries we don’t even know how many women there are, let alone how poor, or how healthy, or how well educated they are, or what work they do, or how they spend their time. The data revolution must be gendered.
Gendering the data revolution means thinking about gender at two levels. Firstly, of course the data itself. In the IEAG report, we pointed out how ‘gender inequality and the undervaluing of women’s activities and priorities in every sphere has been replicated in the statistical record’.
Currently, data suffer from several gender blind spots. Firstly, too often gender simply isn’t recorded. This makes data much less useful than it could be. Knowing the number of people in a country living under $1.25 a day, for example, is one thing. But if, say 80% of those people are women, then that implies a very different set of problems and solutions to if the split is 50-50 between women and men. If most recorded violence is domestic violence against women, this implies very different policies to if violence is mainly men attacking other men. Gender disaggregated data is needed to make women visible and to make good policy. In this respect, gender is just one of many blind spots in much of the data that are produced – numbers on disability, or ethnicity, for example, are also often lacking but are essential for diagnosing and solving the inequalities and exclusion that drive poverty.
It’s not just women themselves who are invisible. Some of the issues most important to their lives are also uncounted and therefore invisible in policy agendas. Labour market statistics designed by women, for example, would almost certainly take into account time spent on domestic work. Surveys in Kenya, Mozambique and Liberia, for example, found that over half of all women cited childcare responsibilities as a constraint on them achieving their livelihood ambitions. In Kenya the figure was over 80 per cent.
Not measuring the realities of the working lives of half the population will mean worse employment policy. Attempts to increase productivity through, for example, offering workers more training, will be of no use to people whose time is already unacceptably squeezed between the different types of work they are required to do. Employment policy using data collected with a gender lens looks different in significant ways – and the same is true of other areas.
Collecting data that more accurately reflects the experiences of women, and that provides policy makers with the raw materials for better, more gender sensitive, policy, is one part of the gender data revolution. But there is a second – putting data in the hands of women themselves.
The data revolution is not for just more data about people – it’s also more data for people – putting data and information in the hands of women themselves, so they have the tools to demand accountability. In many countries, women have less education, and, when they have the chance to specialise, are less likely to do maths, economics or computing than their male counterparts. If new technology is one of the defining characteristics of the data revolution then women are lagging behind there too – in Africa, Asia and Latin America women’s mobile phone ownership is less than men’s, often a lot less.
How can the data revolution be a gender data revolution? There are two priorities
- Women need to become more visible in data. This means collecting more data, through well-functioning civil registration systems, it means disaggregating all data by gender as a matter of course, and it means collecting data on the things that matter most to women’s lives.
- Women need to become active users of data. Many already are. But data literacy programmes must make a special effort to reach out to girls and women, compensating for the often unstated social norms that reserve maths, computing and similar disciplines for boys and men.
There is much hype and hope around the data revolution. For it to be made real, the revolution needs initiatives like Data 2x, showing, again and again, that without paying attention to half the world’s population, the hype will be just hype and the hope will not be realised. The data revolution must be gendered, can be gendered, and will be gendered.
4 Comments